Enhancing N-BEATS Model with LSTM Encoders for Improved Time Series Forecasting
A novel architecture that induces the concept of recurrent layers in NBEATS model to achieve state of the art results.
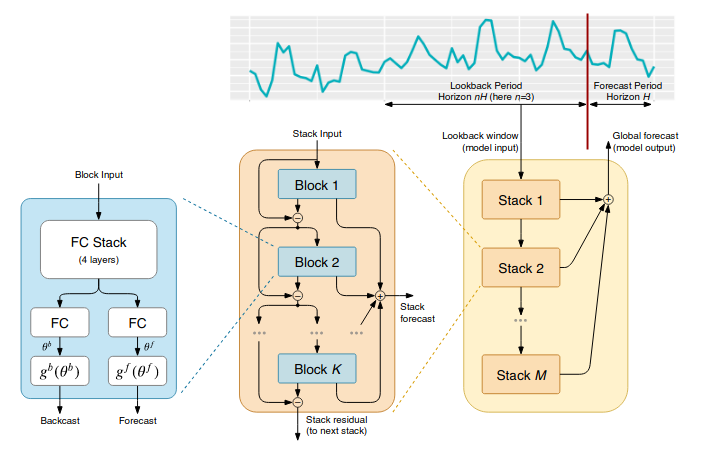
The N-BEATS model has gained significant attention in the field of time series forecasting due to its effective representation learning capabilities using stacked blocks of Multi-Layer Perceptrons (MLPs). In this research project, we propose a novel approach to further enhance the performance of the N-BEATS model by introducing Long Short-Term Memory (LSTM) encoders. By incorporating LSTM encoders, we aim to leverage their ability to capture long-term dependencies and temporal patterns in time series data.
Our proposed architecture combines the strengths of both N-BEATS and LSTM, where the LSTM encoders serve as the backbone for encoding the sequential information. This integration enables the model to effectively capture the complex dynamics and intricate patterns present in time series data, leading to improved forecasting accuracy. Through extensive experimentation and evaluation on diverse real-world datasets, we demonstrate that our enhanced N-BEATS model with LSTM encoders achieves state-of-the-art results compared to the original N-BEATS model and other existing forecasting methods. The incorporation of LSTM encoders provides the model with enhanced temporal modeling capabilities, allowing it to capture both short-term fluctuations and long-term trends in time series data. Overall, our research presents a novel architecture that introduces LSTM encoders into the N-BEATS framework, effectively combining the strengths of both models for more accurate and robust time series forecasting.